Exploring the principles
of human behavior
based on the information-processing
mechanisms of the brain
Humans are subjects who make decisions and learn while interacting with their environment, and their behavior reflects the characteristics of humans and their environment. To understand humans, we construct behavioral models based on the information-processing mechanisms of the brain and verify them using experimental methods to explore the principles of human behavior and conduct educational research on social applications.
How to join
our lab
- As a graduate student
- Lab Visit
Lab tours are always welcome. You can find more information in the "Daily Campus Tour." Our laboratory is available for both online and on-site visits. - Lab Experience
If you want to learn more about our research, we also offer "Lab Experience." This is for those who are considering entering our lab. The schedules and content of the Lab Experience are to be announced. We prepare a 1~2 week program consisting of lectures on reinforcement learning models of decision-making and practical training in behavioral experiments.
- As a researcher
- Now we are looking for a postdoctoral researcher (http://www.naist.jp/en/about_naist/other_information/job_opportunities/230405_2.html) Please contact us if you are interested in this position. We also accept Postdoctoral Fellows (PD) from the Japan Society for the Promotion of Science (JSPS). Please contact us for more information.
Research
Procedures
- Research
- We combine computational approaches using computational models of learning, such as reinforcement learning, and experimental approaches using human subjects.
We also use data-driven approaches using data from large neuroimaging/behavioral databases. Please refer to "Research Topics" below for more detailed information. Neural measurements using EEG and MRI are conducted at ATR Brain Information and Communication Laboratories. ATR is located about 15 minutes by car from NAIST.
- Supervision
- First, students are required to read previous studies on the research theme of their interest and take time to decide on a research theme. Once the theme is decided, we will surpervise to plan experiments, analyze data, summarize the results, and present the results. Especially in the case of research themes involving neuroimaging experiments, we will spend time on training experimental techniques and data analysis methods.
- Organization
- We collaborate with Mathematical Informatics Lab (Prof. Kazushi Ikeda). Lab seminars are held jointly. Associate Prof. Tanaka is also the head of the Department of Neural Computation in Decision-Making (NCD) at ATR Brain Information and Communication Research Laboratories. Our lab works closely with the members of ATR.
Research Topics
-
1.Neural mechanisms of delay discounting
Reinforcement learning, a theoretical framework in which optimal behavior is learned by trial and error through interaction with the environment, has been proposed as a computational model of decision-making in animals, including humans. To elucidate the neural mechanisms involved in reward prediction, we conducted fMRI, a non-invasive functional brain imaging technique, on healthy participants and analyzed behavioral and brain activity data in a Markov decision problem for long-term and short-term reward prediction using a reinforcement learning model. The results revealed that the ventral part of the cortico-striatal circuit is involved in the prediction with a large discount rate, and the dorsal part is in the prediction with a small discount rate, indicating that the brain performs reward prediction with different discount rates in parallel.
Tanaka, S. C., Doya, K., Okada, G., Ueda, K., Okamoto, Y., & Yamawaki, S. (2004). Prediction of immediate and future rewards differentially recruits cortico-basal ganglia loops. Nature neuroscience, 7(8), 887–893. https://doi.org/10.1038/nn1279
-
2.Delay discounting and serotonin
Previous studies have reported that serotonin, a brain substance, is involved in time discounting, mainly in animal experiments using rats, but consistent results have yet to be obtained in humans. To investigate the relationship between discount rates and serotonin, we developed an intertemporal choice task in which participants choose between a small immediate reward and a large, time-consuming reward and measured and analyzed their behaviors and brain activities during the task while artificially adjusting the level of serotonin in their brains. The results showed that when serotonin levels were normal, multiple discount rates of reward prediction were distributed from ventral to dorsal regions of the striatum, which is consistent with previous studies. On the other hand, we found that the ventral part of the striatum was predominant when serotonin was low, and the dorsal part of the striatum was predominant when serotonin was high, indicating that the activity of the striatum is affected by the action of serotonin.
Tanaka, S. C., Schweighofer, N., Asahi, S., Shishida, K., Okamoto, Y., Yamawaki, S., & Doya, K. (2007). Serotonin differentially regulates short- and long-term prediction of rewards in the ventral and dorsal striatum. PloS one, 2(12), e1333 https://doi.org/10.1371/journal.pone.0001333
-
3.Altered delay discount and problematic behavior
Delay discounting is a topic of economics study and has recently been pointed out to be related to social and health problems such as multiple debts and obesity. It has also been used as a model of impulsivity, a symptom of psychiatric disorders, and a behavioral trait of adolescents. Therefore, we clarify the relationship between the parameters of the decision-making model and problematic behaviors and contribute to the understanding and preventing social and health problems. In response to an economic study showing that people with a high discount rate and no asymmetry in the discount rate between rewards and losses (sign effect) have higher rates of multiple debts and obesity, we conducted an experiment to elucidate the brain mechanism of the sign effect. In an experiment using the intertemporal choice between rewards and losses task, we estimated the discount rate from the subjects' behavioral data and compared the brain activities of the groups with and without the sign effect (asymmetry of the time discount rate between rewards and losses in behavior). The results suggest that the asymmetry of reward and loss in striatal activity is caused by the sign effect, i.e., the asymmetry of reward and loss in behavior.
Tanaka, S. C., Yamada, K., Yoneda, H., & Ohtake, F. (2014). Neural mechanisms of gain-loss asymmetry in temporal discounting. The Journal of neuroscience : the official journal of the Society for Neuroscience, 34(16), 5595–5602. https://doi.org/10.1523/JNEUROSCI.5169-12.2014
-
4.Temporal credit assignment and serotonin
The impulsive choice to frequently select "small immediate rewards" in intertemporal choice occurs when the weight of delayed rewards is reduced when the discount rate is high, but it can also occur when delayed rewards obtained in learning are only associated with the immediate past (credit assignment problem). To test this, we estimated the decay rate of subjects' temporal credit assignment from their behaviors in a learning task in which there is a temporal delay between the past action and the current reward under the serotonin level is manipulated. We found that the decay rate of the temporal credit assignment to the loss was larger when the serotonin level was decreased than in the normal condition. These results suggest that serotonin is involved in both the future time scale (discount rate of reward prediction) and the past time scale (decay rate of temporal credit assignment).
Tanaka, S. C., Shishida, K., Schweighofer, N., Okamoto, Y., Yamawaki, S., & Doya, K. (2009). Serotonin affects association of aversive outcomes to past actions. The Journal of neuroscience : the official journal of the Society for Neuroscience, 29(50), 15669–15674. https://doi.org/10.1523/JNEUROSCI.2799-09.2009
-
5.Data-driven approach
Computational psychiatry has gained momentum, in which the mechanisms of psychiatry disorders are understood as computational models by applying the hypothesis-testing approach to patients with psychiatry disorders, as described in topics 3 and 4 above. On the other hand, the data-driven approach, which combines multivariate brain and behavioral data with machine learning, is attempting to understand the mechanisms of psychiatry disorders and individual characteristics in a "holistic" manner without being bound by any specific hypothesis. Examples of such approaches include identifying disorders using resting-state functional connectivity patterns, biotype identification, and prediction of individual characteristics. However, data-driven analysis with high accuracy and generalization performance requires a large amount of data and the development of algorithms to extract features successfully. The applicant has elucidated the brain network common to various types of anxiety by using large data sets and practical machine learning algorithms tailored to the characteristics of each data set. By extracting features from brain activity data reflecting different types of anxiety, i.e., brain activity during anxiety-provoking tasks and resting-state brain activity related to daily anxiety characteristics, and reducing the dimension of the features, we identified the brain network common to these types of anxiety. We developed a method for identifying patients with anxiety-related diseases from this brain network. We confirmed that it is possible to discriminate patients with anxiety-related disorders from this brain network.
Takagi, Y., Sakai, Y., Abe, Y., Nishida, S., Harrison, B. J., Martínez-Zalacaín, I., Soriano-Mas, C., Narumoto, J., & Tanaka, S. C. (2018). A common brain network among state, trait, and pathological anxiety from whole-brain functional connectivity. NeuroImage, 172, 506–516. https://doi.org/10.1016/j.neuroimage.2018.01.080
-
6.Computational model of obsessive-compulsive disorder
We use computational models to describe human behavior and investigate the relationship between the parameters, individual characteristics, and neural mechanisms. Specifically, we are constructing computational models of psychiatric disorders and validating them with empirical data. We model obsessive-compulsive disorder (OCD) symptoms as implicitly learned maladaptive behaviors. Simulations in the reinforcement learning framework show that agents implicitly learn to respond to intrusive thoughts when the memory trace signal for past actions decays differently for positive and negative prediction errors. Moreover, this model extends our understanding of the therapeutic effects of behavioral therapy in OCD. Using empirical data, we confirm that patients with OCD show extremely imbalanced traces, which are normalized by serotonin enhancers. We find that healthy participants also vary in their obsessive-compulsive tendencies, consistent with the degree of imbalanced traces. These behavioral characteristics can be generalized to variations in the healthy population beyond the spectrum of clinical phenotypes.
Sakai, Y., Sakai, Y., Abe, Y., Narumoto, J., & Tanaka, S. C. (2022). Memory trace imbalance in reinforcement and punishment systems can reinforce implicit choices leading to obsessive-compulsive behavior. Cell reports, 40(9), 111275. https://doi.org/10.1016/j.celrep.2022.111275
-
7.Large-scale neuroimaging database
Recently, the reproducibility of results of scientific research has become a major issue in scientific fields as a whole, and various attempts have been made to ensure reproducibility in brain research. Among them, large-scale data collection and sharing are considered the most important to ensure sufficient statistical power. As a member of the multi-institutional human brain MRI large-scale database project, we have constructed a globally valuable multicenter and multi-disease brain MRI database that is publicly available.
Tanaka, S. C., Yamashita, A., Yahata, N., Itahashi, T., Lisi, G., Yamada, T., Ichikawa, N., Takamura, M., Yoshihara, Y., Kunimatsu, A., Okada, N., Hashimoto, R., Okada, G., Sakai, Y., Morimoto, J., Narumoto, J., Shimada, Y., Mano, H., Yoshida, W., Seymour, B., … Imamizu, H. (2021). A multi-site, multi-disorder resting-state magnetic resonance image database. Scientific data, 8(1), 227. https://doi.org/10.1038/s41597-021-01004-8
-
8.Development and Validation of Mathematical Models of Pain and Analgesia
To appropriately regulate bodily functions, humans must continuously and accurately interpret sensory information. However, when it comes to pain, the brain often forms erroneous beliefs, leading to an overestimation of pain intensity. In this study, we developed a novel computational model grounded in Bayesian theory, in which beliefs and perceptions about pain are interactively updated. Using this model, we demonstrate how incorrect beliefs and perceptions regarding pain can form in a mutually reinforcing manner. Simulating pain perception with this model successfully reproduced a perceptual phenomenon known as offset analgesia—a marked reduction in perceived pain following a brief, minor fluctuation in pain intensity. This finding suggests that the phenomenon known as self-fulfilling pain, in which pain intensity changes in the direction of one’s expectation, arises through a process of disambiguating uncertain perceptual inputs. Importantly, this unified model explains offset analgesia without the need to invoke a special analgesic mechanism such as the traditionally proposed temporal filter. Thus, our model offers a new theoretical framework that unifies the understanding of pain and analgesia along a single perceptual dimension. Furthermore, simulations adjusting parameters corresponding to attention, hypervigilance, and sensory monitoring were also conducted. These allowed us to examine anomalies in analgesic processing observed in individuals with chronic pain conditions.
Ogishima H, & Tanaka SC. Constructing a Computational Model of Pain Based on the Free Energy Principle: Realizing the Interaction Between Beliefs and Perceptions. NEURO2024, 2024 (submitted).
-
9.Modeling Decision paralysis with Mixed Reverse-Forward Kullback Leibler divergence
This study presents a computational model of decision-making based on Reinforcement Learning (RL), designed to han- dle situations where multiple options are equally viable. The model modifies the policy improvement process by using both the forward and reverse Kullback-Leibler (KL) divergences: forward KL (FKL) averages over all options (mean- seeking), while reverse KL (RKL) favors high-valued options (mode-seeking). Based on the self-analysis of executive function difficulties by an individual with autism spectrum disorder (ASD), the model explores two types of cogni- tive saturation: saturation of meaning, where goals are unclear despite proper perception, and saturation of affordance, where goals are clear, but not the action. Both saturations lead to cognitive overload and to the eventual freezing of the decision-making process. By separating the decision-making process into intentions (goals) and actions (affordances), the proposed model offers a structured framework for understanding how cognitive overload occurs. It posits that freez- ing is driven by the mean-seeking nature of FKL divergence, which overwhelms the cognitive system when too many options are available, resulting in decision paralysis. The proposed model may clarify the cognitive challenges faced by individuals with ASD, particularly when multiple options are present. It also highlights the potential of RL-based models to explain these phenomena, offering a structured approach to understanding the interaction between goal selection and action execution. This study hopes to provide tools for the development of solutions for those who frequently expe- rience cognitive ”freezing”, by altering the nature of the problem itself to remove the source of the issue. Ultimately, this model may inform future research and contribute to strategies aimed at mitigating decision-making difficulties caused by cognitive overload.
Ilboudo WEL & Tanaka SC. Kullback-Leibler Divergence for Modeling Intent and Affordance Saturation in Reinforcement Learning. Reinforcement Learning and Decision Making (RLDM) 2025, 2025.
Member
Professor
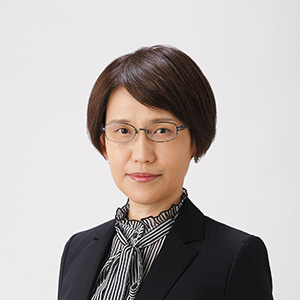
- 田中 沙織 Saori C. TANAKA
- Link
ResearchmapGoogle Scholar
Assistant Professor
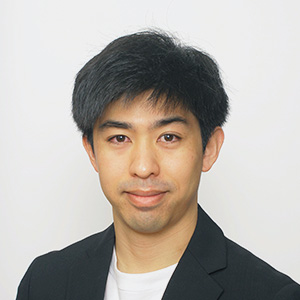
- 荻島 大凱 Hiroyoshi OGISHIMA
- Link
Researchmap
Specially Appointed Assistant Professor
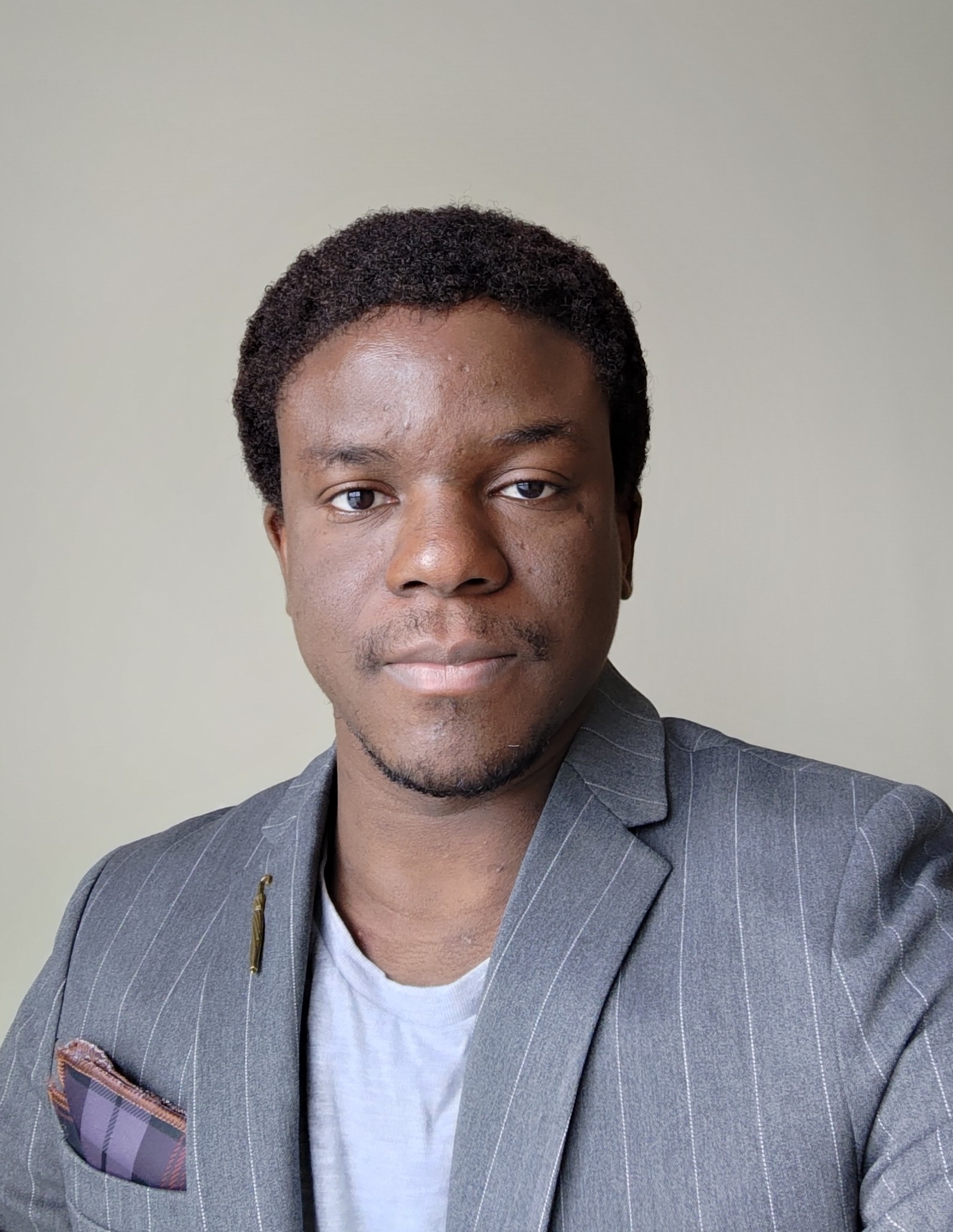
- Wendyam Eric Lionel Ilboudo
- Link
Google Scholar
Visiting Assistant Professor
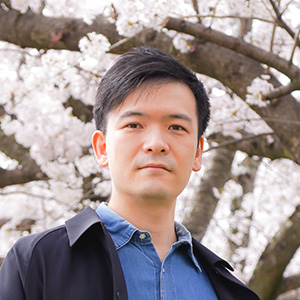
川島 一朔
Issaku KAWASHIMA, ATR
Commissioned Faculty Member
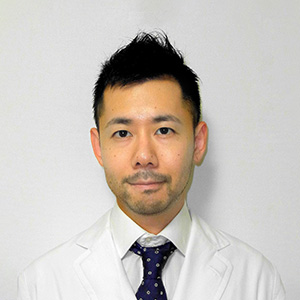
酒井 雄希
Yuki SAKAI, ATR
Postdoctoral Fellow
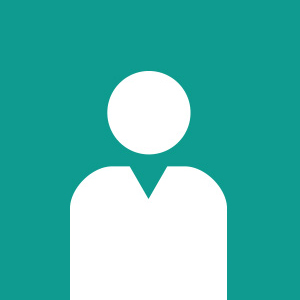
堀之内 峻之
Takayuki HORINOUCHIPD (JSPS)
Students
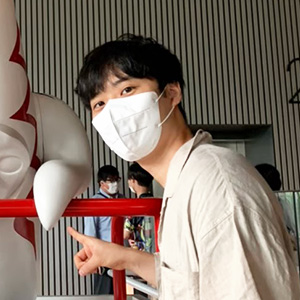
佐野 海士
Kaito SANOD2
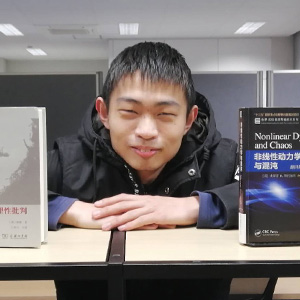
Gao YulunD2
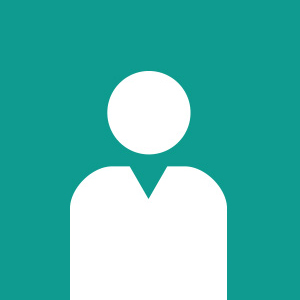
大西 達也
Tatsuya ONISHIM2
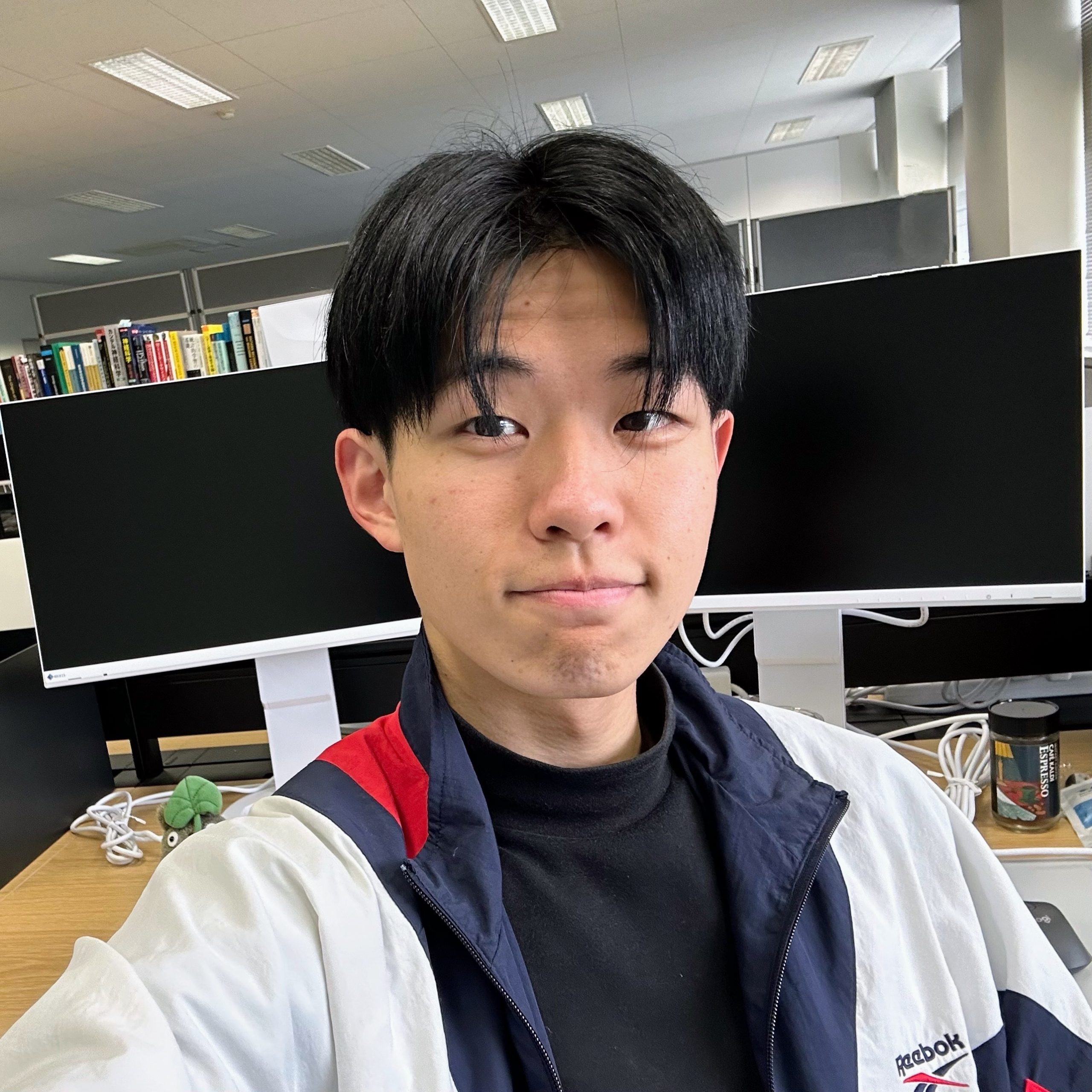
白川 琢磨
Takuma SHIRAKAWAM2
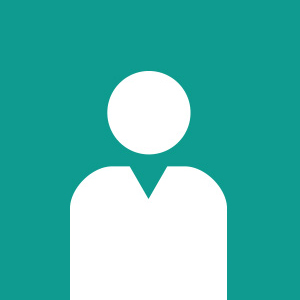
成田 大祐
Daisuke NARITAM2
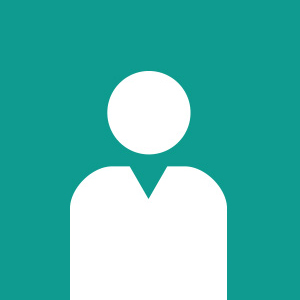
西谷 香紀
Kano NISHITANIM2
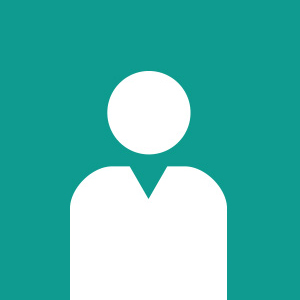
児玉 創太郎
Sotaro KODAMAM2
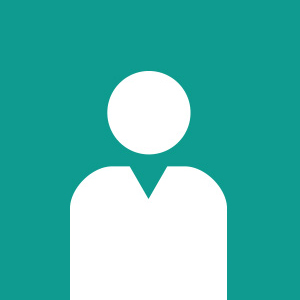
渡邉 奈緒美
Naomi WATANABEM2
Alumni
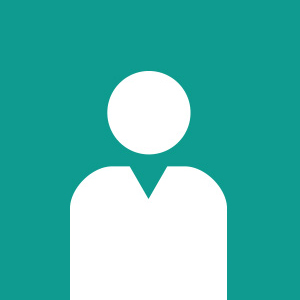
Erik HAINZLMEIER
Technical University of Munich
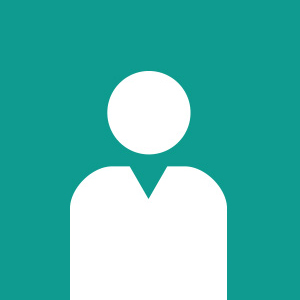
小池 教
Minori KOIKE
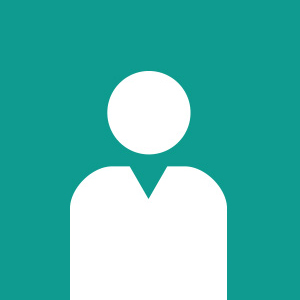
中川 綾子
Ayako NAKAGAWA
Publications
- Journal
Please refer to Researchmap.
https://researchmap.jp/xsaori/published_papers
Contact
Please contact us from here -> Google form will open
Location:
Computational Behavioral Neuroscience Laboratory Division of Information Science
Nara Institute of Science and Technology (6th floor, Building B, Division of Information Science)